Start your project with Spark
Find a solution that's right for your business, on your terms.
Thank you! Your submission has been received!
Oops! Something went wrong while submitting the form.
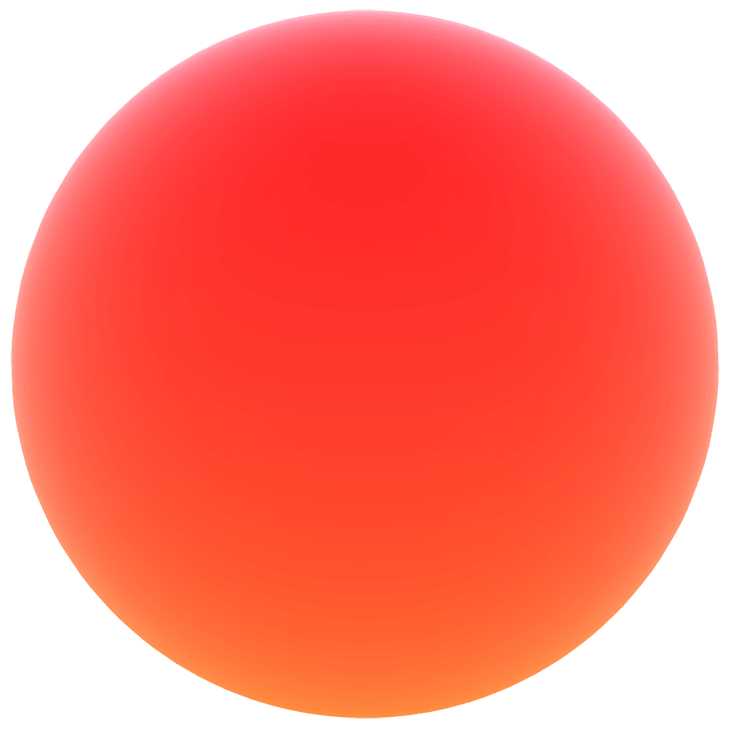
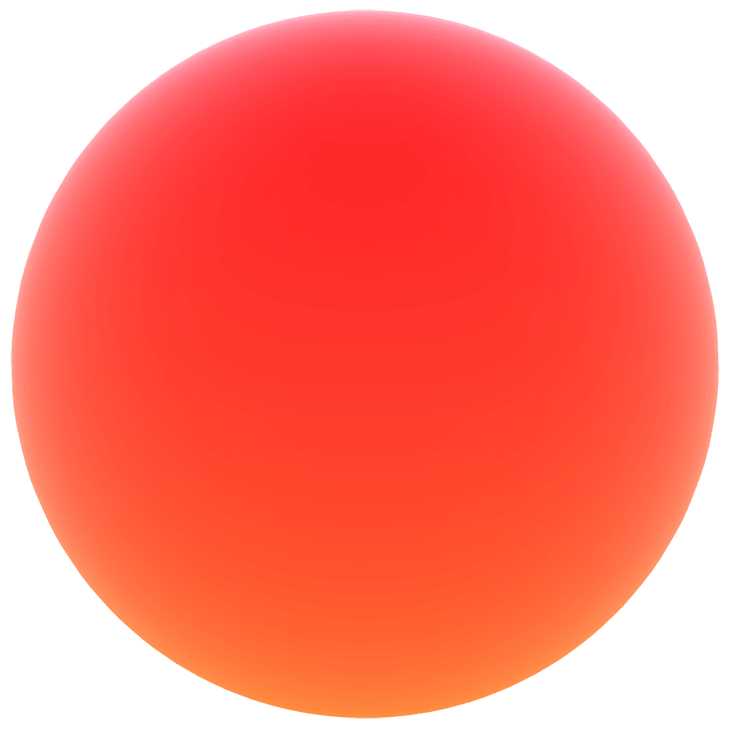
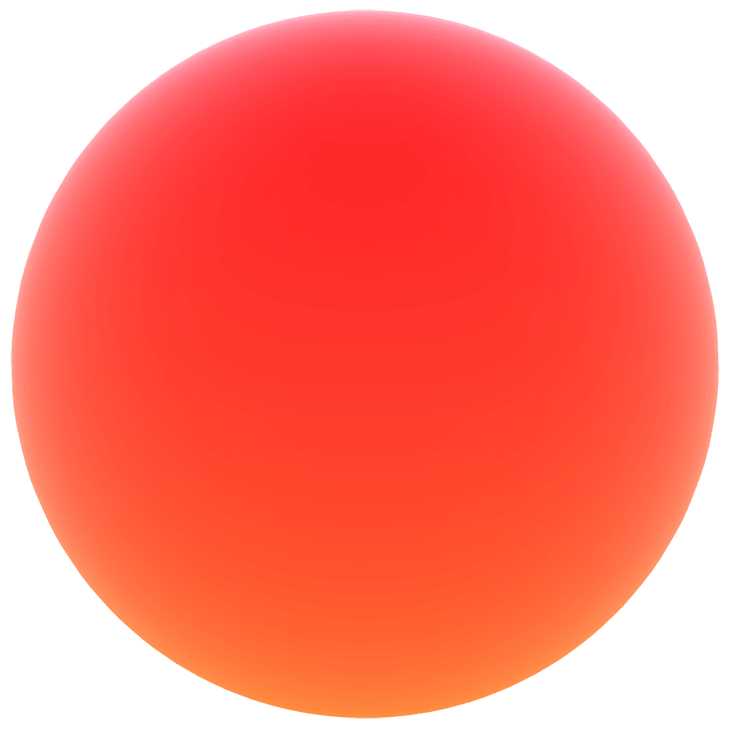
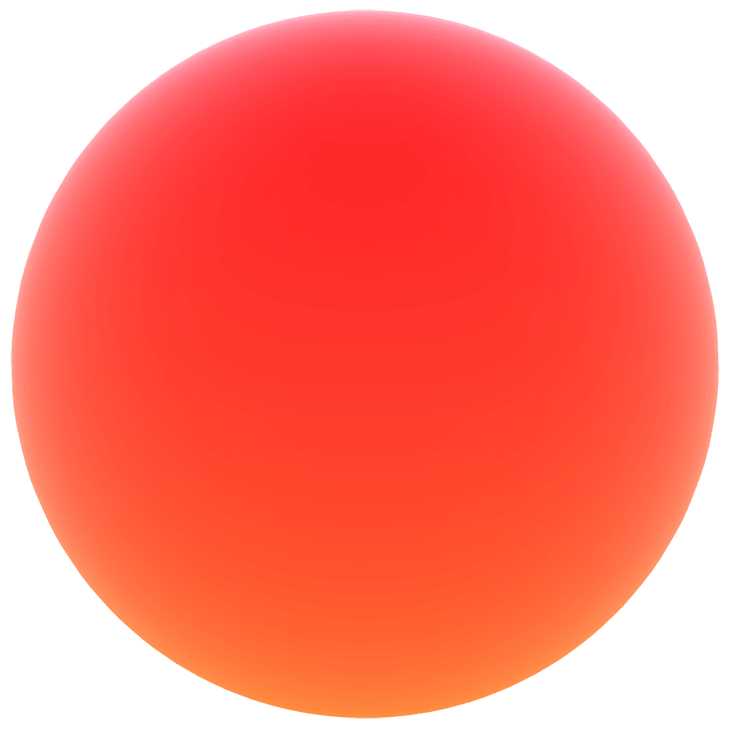
Data is king, but there is so much of it out there that it can be hard to rule it without the right architecture. And the trouble isn't just finding the right architecture, but adapting from your original data structure, unwieldy as it may currently be, to something that gets data into decision-makers hands faster and more democratically. It can be daunting. The 2021 data landscape is like another planet compared to what it looked like three decades earlier. Instead of centralized data silos or newer "data lakes," a major shift is underway toward the "data mesh" architecture. While it's a trendy buzzword, you've probably been hearing a lot of, what is it exactly? As you grapple with becoming a more data-driven organization, it's essential to find the right solution. So, let's talk about what data mesh is, what it isn't, and how it could be the key to solving your data challenges.
Let's backtrack a little to help explain what data mesh is with a concept we've talked about several times before—microservices architecture. Another way to think of this is a "services mesh" where a monolithic software application is deconstructed into separate, independent services that respond to each other and deliver a host of separate services. One of the key difficulties for microservices has been how they interact with data that still lives in giant silos or "lakes." The need for a new data architecture emerged.
Simply put, data mesh is the data platform version of microservices. The term was first coined by Zhamak Dehghani. Instead of housing data in lakes and silos that can be difficult for a growing number of cloud apps to access across environments, a data mesh platform is more like connective tissue providing people with access data across software environments through a domain-oriented, self-serve data design. This is a fresh way of thinking about data structures corresponding to Eric Evans' data-driven design theory. It links your data's language and structure with its business domain, essentially turning the data into a product. As Forbes notes, this helps software developers to create a bridge to connect a centralized application with different on-site data environments.
Developers and organizations alike are excited about data mesh because it makes big data easier to access and analyze in many different situations. This paradigm shift stems from four foundational principles:
With this understanding, it's time to address this new architecture's benefits and its corresponding challenges.
Three decades ago, data warehouses were the norm. Business demands and technology were different back then. Those warehouses required a dedicated group of IT specialists with the technical expertise to keep the data flowing between the warehouse and those who needed it. The analysis took a lot longer, and insight required significant time and energy.
Data warehouses eventually morphed into data lakes, which, although they are better at streaming the data for analysis, enrichment and transformation, are still relatively centralized and create certain undesirable barriers. The central ETL (extract, transform, load) stream isn't flexible enough to provide organizations the control they want over ever-growing data volumes. And as organizations strive to turn themselves into data companies, they require more agility and flexibility in how their data use cases are handled. A central data lake platform still isn't flexible enough for many businesses. Enter data mesh's key benefits:
As wonderful as this is and as many opportunities as this new decentralized data fabric creates, it's not a panacea for every single business scenario. It's important to understand these limitations.
As with any major business decision, it's essential to weigh your needs against both the benefits and drawbacks of any new data architecture.
As the world continues to grapple with and try to emerge from the Covid-19 pandemic, businesses have had to confront new realities, one of which is how crucial it is to transform themselves into more data-driven organizations. Decision-makers list this as a top strategic objective as many companies try to reinvent themselves into more agile and adaptable creatures. How to squeeze the most out of data, now to organize it, how to package it, how to transfer and transform it remains a central concern, and data mesh is one promising direction.
Data mesh empowers companies to use intelligence first and foremost, thinking about how their data packages can create better customer experiences through extreme personalization. At the same time, data-driven optimizations like these can help reduce operational costs while making employees at all levels more powerful by putting trend analysis and business intelligence insight into their hands.
And yet, it's important to remember that data mesh is just one potential path to take on this journey. You must evaluate whether your target goals line up with this new paradigm or if another one would suit your organization better. For that, you can evaluate your answers to the following questions:
Data mesh might fit your criteria if you answered yes to all of those questions, especially the last one. If so, you can start by getting executive support, obtaining a budget, and gathering your team. Members of the domain-based team should include data engineers who understand your need for transformation and are able to envision data-as-a-product, and whose end consumers are decision-makers at all different levels.
You can then cross-train staff members to pass on domain expertise throughout the organization and automate as many data engineering tasks as possible with Infrastructure as a service (IaaS) taking the reins on tactical concerns.
If you're struggling with this question, really consider those last five questions. Data mesh may both excite and intimidate your data officers. As data becomes more distributed and democratized, security concerns arise, which is a fair point for any new architecture. The counterbalance is how data mesh architectures require observability in scalable, self-serve data. Observability is really the only way to own and control your data.
It might help to imagine a data mesh architecture as a sort of federation founded on self-service analytics where business domain experts churn data and publish reports as a data product for the company at large to consume. The disparate, yet connected businesses agree on the basic rules of data exchange. Just remember to ask those five questions above before deciding whether to mesh or not before jumping in. You need to be sure your data is safe and that it will work well within this architecture, and not invest all the time and energy to switch just because it's currently a popular concept. Evaluate whether this approach will bring lasting value and solve real problems before investing the time and money. As ever, base your decisions on the data.
Are you contemplating whether your organization would benefit from data mesh? Don't stress out about it without talking to an expert first. Our data experts can help you reach the right decision, so let's talk about it today.